김현욱 교수님 : Prediction of metabolites associated with somatic mutations…
페이지 정보

작성자 최고관리자
조회 2,573회 작성일 2024-04-08 10:08
본문
Prediction of metabolites associated with somatic mutations in cancers by using genome-scale metabolic models and mutation data
https://genomebiology.biomedcentral.com/articles/10.1186/s13059-024-03208-8
abstract:
Background
Oncometabolites, often generated as a result of a gene mutation, show pro-oncogenic function when abnormally accumulated in cancer cells. Identification of such mutation-associated metabolites will facilitate developing treatment strategies for cancers, but is challenging due to the large number of metabolites in a cell and the presence of multiple genes associated with cancer development.
Results
Here we report the development of a computational workflow that predicts metabolite-gene-pathway sets. Metabolite-gene-pathway sets present metabolites and metabolic pathways significantly associated with specific somatic mutations in cancers. The computational workflow uses both cancer patient-specific genome-scale metabolic models (GEMs) and mutation data to generate metabolite-gene-pathway sets. A GEM is a computational model that predicts reaction fluxes at a genome scale and can be constructed in a cell-specific manner by using omics data. The computational workflow is first validated by comparing the resulting metabolite-gene pairs with multi-omics data (i.e., mutation data, RNA-seq data, and metabolome data) from acute myeloid leukemia and renal cell carcinoma samples collected in this study. The computational workflow is further validated by evaluating the metabolite-gene-pathway sets predicted for 18 cancer types, by using RNA-seq data publicly available, in comparison with the reported studies. Therapeutic potential of the resulting metabolite-gene-pathway sets is also discussed.
Conclusions
Validation of the metabolite-gene-pathway set-predicting computational workflow indicates that a decent number of metabolites and metabolic pathways appear to be significantly associated with specific somatic mutations. The computational workflow and the resulting metabolite-gene-pathway sets will help identify novel oncometabolites and also suggest cancer treatment strategies.
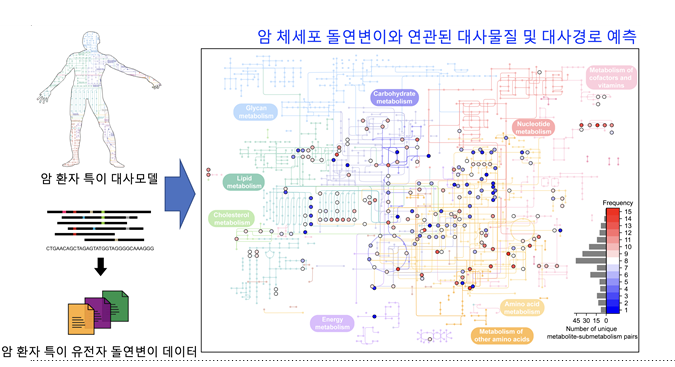